4 minutes
The term “HMDA outlier” is used by the National Credit Union Administration and other regulatory agencies to describe part of their criteria for selecting financial institutions for fair lending reviews. Unfortunately, it can be a daunting task for a compliance officer to get a handle on the CU’s Home Mortgage Disclosure Act data and answer the question: “Is my credit union a HMDA outlier?” This article shares a straightforward methodology, using public HMDA data, to identify HMDA outliers.
NCUA’s 2013 Fair Lending Examination Program and Compliance Assistance states: “Federal credit unions that are HMDA outliers and demonstrate the potential for higher fair lending risk are subject to a fair lending exam in accordance with the FFIEC exam procedures.” In that guidance, NCUA also states that it will review the credit union’s annual HMDA report, and if that review indicates the credit union’s lending practices fall outside the normal range with regard to pricing, denials, withdrawals, or lending terms (when compared to other financial institutions) the credit union will be considered a HMDA outlier.
A Data-based Approach
In keeping with the NCUA guidance, ComplianceTech suggests using a statistics-based approach to determine whether a federal credit union is a HMDA outlier. This approach computes an average score (such as for denial rate, pricing and lending term) for a group of “peer” lenders, and then determines which of the individual lenders’ scores are far enough from the average to be considered an outlier.
In the world of statistics, the standard deviation provides a benchmark for how far from the average a lender’s score can be before it’s considered an outlier. The general rule of thumb is that a lender’s score should be within 2 standard deviations (plus or minus) of the average of its peers’ scores to be considered “normal.” This rule of thumb is useful because statistics suggest that approximately 95 percent of the scores should be within two standard deviations of the average score, assuming the data is normally distributed. Applying this standard statistical approach to an analysis of the public HMDA data enables credit unions to see how they perform compared to other lenders.
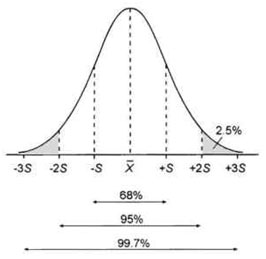
Figure 1 shows an illustration to help explain. The middle point (the average) is labeled “X” and each Standard Deviation is labeled “S”. This curve, and the percentages below it, show the likelihood that a score will fall within one standard deviation (68 percent), two standard deviations (95 percent) or three standard deviations (98 percent) of the average.
As an example, using a statistical approach, a credit union may be interested in determining whether it is a HMDA outlier with regard to denial rates (overall or for a particular subset of borrowers). To answer this question, the CU would determine what the individual denial rates are for each lender in a peer group, compute the average denial rate for the peers, and then compute the standard deviation of the peer denial rates. Finally, the CU would compare its denial rate to the average. If the CU’s denial rate is more than 2 standard deviations above or below the peer average, it is considered a HMDA outlier. This approach can be used to determine whether the credit union would pop up on NCUA’s radar as a HMDA outlier for denial rates.
Where Do I Begin? An Exploratory Example
The following example goes a bit deeper.
Table 2 and Table 3 show examples of analysis providing all the information required to determine which lenders are denial rate HMDA outliers in the Washington, D.C., metropolitan service area. Table 2 shows HMDA outliers based on all lenders, while Table 3 shows HMDA outliers based on NCUA lenders only. Note that the complete reports show each denial rate for all the lenders in the peer group, the average denial rate, the standard deviation, and the lenders whose denial rates are more than two standard deviations above the average.
Table 2: 2013 HMDA Outlier Analysis–Denial Rate Among all Lenders in Washington, D.C., MSA

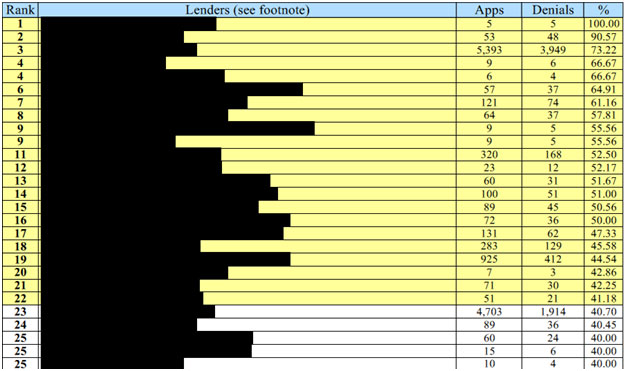
Table 3: 2013 HMDA Outlier Analysis–Denial Rates–Among NCUA Lenders in Washington, D.C., MSA

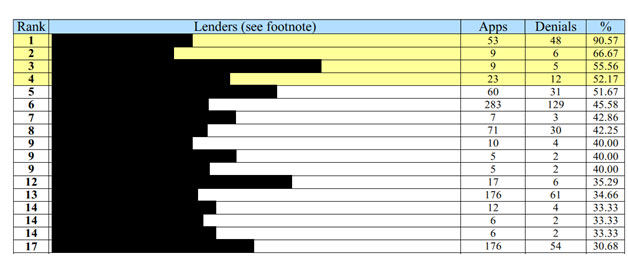
The denial rates in yellow are more than two standard deviations higher than the average and, therefore, these lenders would be considered HMDA outliers for denial rates. Subsequently, the lender may choose to do a deeper review of denial rates and see whether it occurs with a particular group of borrowers.
The examples above focus on overall denial rates. Remember that there are many other ways to review the data for outliers, including pricing and denial disparities. Each of these tests can be performed with “drill down” to see if there is anything suggesting differences in lending based on race, ethnicity and/or gender.
It’s worthwhile to take a statistical look now at whether your most recent data submission suggests your credit union is a HMDA outlier compared to the 2013 aggregate data—and then confirm your findings with a second statistical analysis when the new, aggregated peer data is released in September.
Dana Ginsburg manages customer relations at ComplianceTech. She advises and trains clients on how to analyze data using automated fair lending software solutions.